Having a website and web-based communication media (social networks, blogs, etc.) is all well and good, but you still need to make sure you use them effectively to increase your visibility and sales effectiveness.
Every post, every text, every photo, every comment on social networks or a website is used by algorithms and can be referenced using words. Equipping yourself with the means to understand and predict the results of your SEO[1] efforts is now fundamental, especially if your site is at the centre of your marketing system.
While optimising search engine optimisation has always been one of the challenges of web communication, statistical methods and the data ecosystem are now opening up new potential for analysis through Data-Driven SEO.
Automation, reporting and alert systems are at the heart of these new approaches. These tools have become essential for improving responsiveness.
A Data Scientist can help to understand search and traffic data, automate analyses and predict the results of SEO strategies. How do they work? What answers are they helping to build?
[1] SEO: Search Engine Optimization. SEO is the term used to define all the techniques used to improve the visibility of a website on search engine results pages. It is also known as natural referencing.
1. SEO at its simplest
Search engines have crawlers, also known as spiders. In practical terms, these are computer programmes that crawl different sites in order to retrieve page content. This content is then analysed and indexed. When a user performs a search on a search engine, it is these indexes that are queried. The search engine then proposes the most relevant response (combination of indexes) to the user’s query by displaying the results on the SERP[1].
The criteria used by search engines to give preference to a result are numerous. Google is said to use more than 200 of them – although not all of them are equally important. These include
- the age of the domain
- site structure
- page load speed,
- content quality,
- the uniqueness of the content,
- relevance and number of inbound/outbound links…
Most SEO approaches are based on empirical optimisation methods.
[1] SERP: Search Engine Result Page
2. Available data and the contribution of the Data Scientist
In the context of SEO, a Data Scientist can help to better understand search and traffic data, enable ‘real-time’ optimisation research and predict the results of SEO strategies. To do this, they have a number of analytical tools at their disposal.
Web page optimisation
In the context of SEO, a Data Scientist can help to better understand search and traffic data, enable ‘real-time’ optimisation research and predict the results of SEO strategies. To do this, they have a number of analytical tools at their disposal.
Web page optimisation
Logs are the computer traces left behind when a page is visited. The work of the data scientist will enable prioritisation of pages to be closed or redirected, automated analysis of log files, detection of trend breaks and error peaks. Data visualisation approaches can be used to provide results in the form of an alert system or dashboard.
The preliminary analysis will enable the data to be classified, distinguishing between visits by crawlers, search engine indexing and user visits. Google’s crawlers, as well as its competitors, have user-agents with predefined IP addresses, which enable them to be identified in the mass of visits.
The logs can then be studied using various approaches:
- highlight pages returning errors (404 error for a deleted page, etc.),
- detect pages with abnormally long loading times,
- or tracking pages that have had few or no visits in relation to the crawl time they consume.
It is better to save this crawl time for other pages with higher added value.
Based on the site’s page inventories and structure, we can better analyse the reasons for the performance of certain pages. Using a few queries, the data scientist can isolate pages that are orphaned or poorly linked.
Marketing Intelligence
It is now possible to anticipate the possible results of a search engine optimisation policy. Data can be used to monitor the emergence of a competitor, new trends or even new products.
Web scraping methods can be used to automate the journey through a website. In the same way as search engine crawlers scour the sites to be referenced, it is possible to frequently retrieve the SERP for a list of predefined keywords.
This gives the data scientist multiple avenues of analysis: he can analyse positions at a certain point in time, but also temporal variations by historising ranking data. With a bit of inventiveness, he can propose a control chart and put in place management rules with the SEO experts so as to be alerted as quickly as possible in the event of an incident on the site. By counting, they can see the emergence of a competitor.
In addition, the Data Scientist will be able to automate and industrialise certain tasks in the search for new keywords and to record some of these keywords. They can be used to highlight new services or products, differentiating factors through the creation of new pages on the site, or simply to create a pool of future opportunities. Just think of a keyword like “external phone battery” and the websites that sniffed it out first.
Decision-making tools
Audience tracking tools, such as Google Analytics or Matomo, give data scientists access to all the data they need to create dashboards that facilitate decision-making and responsiveness.
They will be able to create customised graphs based on tracking user behaviour and facilitating adhoc developments in commercial environments in particular. In this way, users can be tracked page by page, or even within each page. For an e-commerce site, the Data Scientist will create a conversion tunnel and provide an understanding of what happens at each stage of the transaction by cross-referencing visitor data with logs, for example.
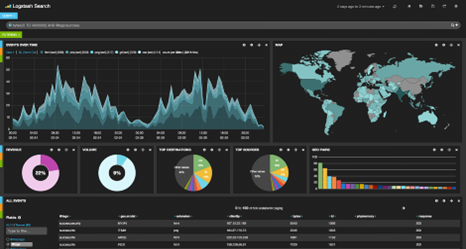
3. Tools and methods to take content analysis further
There are many tools available to data scientists for analysing SEO. In addition to the off-the-shelf solutions offered by publishers, Open Source tools and languages can cover the whole range of needs mentioned above.
For example, the ELK suite (ElasticSearch, Logstash, Kibana) brings together a log analysis tool (Logstash), an indexing solution (Elasticsearch) and the associated data visualisation (Kibana).
Data Science languages, including Python and R, both of which can be used to perform queries to retrieve pages or information from the Internet, offer methods for temporal analysis, libraries dedicated to data visualisation, and so on. Through packages such as RGoogleAnalytics and searchConsoleR, R can even be used to retrieve certain data automatically using Google APIs.
These two languages, and Python in particular, also offer excellent natural language processing (NLP) tools. The data scientist can, for example, propose a method for detecting duplicate content, or a syntactic analysis of the best referenced content. Python’s nltk or spaCy packages will be ideal allies in this task.
4. Outlook and future developments
The complexity and opacity of Google’s ranking algorithm make statistical analysis complex. SEO is a constantly evolving field. Building flexible, responsive analysis tools has become essential to meet the need to adapt constantly to complexity and change. The following developments are to be expected and will need to be integrated to continue to ensure the competitiveness of commercial organisations and their communications:
Increasing complexity of factors
Design imperatives
-
Content quality
The growing importance of semantic analysis in search engines, and therefore the need for high-quality, unique and accurate content, especially if the sector is technical and niche.
-
User experience
Increasing emphasis on the user experience, making it imperative for companies to create a website that is user-friendly, easy to navigate and accessible.
In short, companies that want to maintain or improve their ranking in search results will have to adapt to these constantly evolving trends.
Given the complexities involved, the role of the data scientist and the integration of AI may become increasingly central.
Sources: https://www.seo.fr – https://www.orixa-media.com – https://www.blogdumoderateur.com/seo-outils-ia-revolutionner-redaction-contenu/ – https://support.google.com/webmasters/answer/9128668?hl=fr